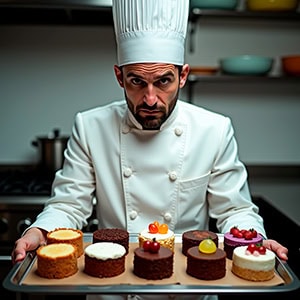
The lying cook
The binomial distribution is used when we want to calculate the probability of obtaining a certain number of successes in a series of Bernoulli trials, assuming we already know the probability of success in each trial. In contrast, the beta distribution is used in the opposite situation: we have observed a given number of successes and failures, and we want to estimate how likely each possible value of the success probability is. In other words, it allows us to update our beliefs about that probability based on the data we have collected.