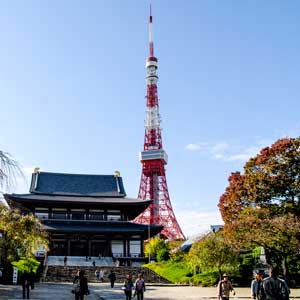
King Kong versus Godzilla
Critical appraisal of ecological studies. Ecological studies are those in which the study population are grouped subjects, so the level of inference is also aggregated.
Evidence Based Medicine pills
Evidence Based Medicine pills
Critical appraisal of ecological studies. Ecological studies are those in which the study population are grouped subjects, so the level of inference is also aggregated.
Ecological fallacy. The ecological fallacy consists in assuming, within an ecological study, that the effect observed at the global level is maintained at the individual level.